Approximate Reasoning for Stochastic Markovian Systems
Complex systems that combine artificial (software-based) components and natural components are the new challenges today in Engineering and Technology. They can be found in areas as diverse as aerospace, automotive engineering, chemical processes, civil infrastructures, energy, healthcare, manufacturing, transportation, and consumer appliances.
When we analyse these systems, we often represent them as stochastic processes to model ignorance, uncertainty or inherent randomness. The stochastic processes are mathematical models that use probabilistic reasoning to abstract the missing information. The goal is to be able to understand when the properties observed on models can be true about the modelled system.
To do this, the project will study the logical and mathematical foundations of an approximation theory for stochastic systems that formalizes this knowledge transfer and supports the process of modelling and simulation of complex systems. This research is multidisciplinary, combining knowledge from Logic, Topology, Measure Theory, Metric Spaces, Transition Systems as well as applied Computer Science and Engineering; the results are expected to have a significant impact in all these fields.
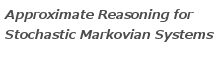